Workshop "Vistas in Control" September 10-11, 2018
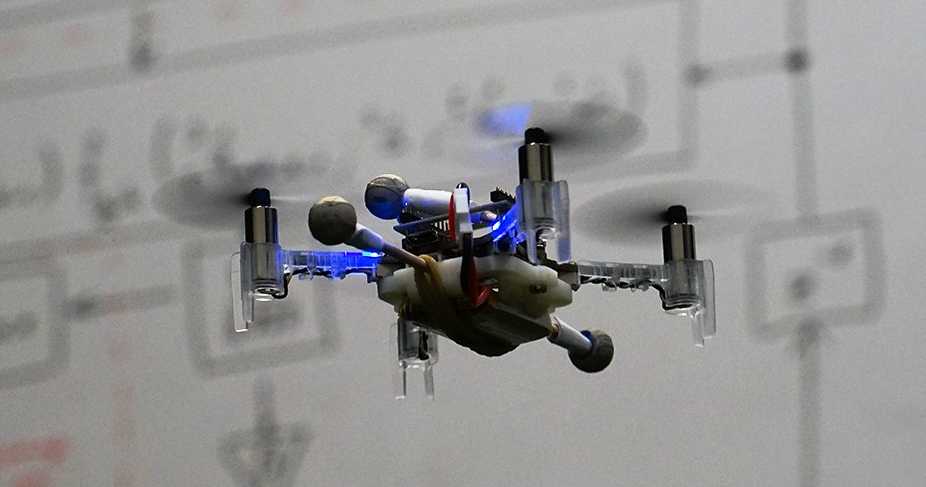
On the occasion of the 50th birthday of the Automatic Control Laboratory, we organized an international workshop to give past and current IfA members the opportunity to attend presentations by leaders in our field, meet old friends, and make new ones.
The workshop titled Vistas in Control was held in Zurich on September 10-11, 2018 at ETH Zurich, Rämistrasse 101, AudiMax HG F30.
It is focused on future directions in control, and we think will provide a great opportunity to identify the main current and future challenges in our field. We have assembled an impressive list of keynote speakers in control systems, related fields (including optimisation, learning, and methods from theoretical computer science), and a range of application areas (including energy, traffic, and robotics). The workshop will emphasize discussion, interaction, and making new connections. In addition to the technical program we will therefore also organize activities to foster informal interactions, among others a banquet where we will recall the history of the institute.
From 19:00 (with registration only): Apéro and banquet dinner at the external page Restaurant Lakeside, Bellerivestrasse, Zürich. The banquet will include retrospective speeches by former ETH Automatic Control Laboratory heads Manfred Morari and Mohamed Mansour and IFAC president Frank Allgöwer.
Tuesday, September 11, 2018, ETH main building, AudiMax HG F30
From 17:45: Farewell reception and concluding discussions break in the restaurant Dozentenfoyer, J floor, ETH main building.
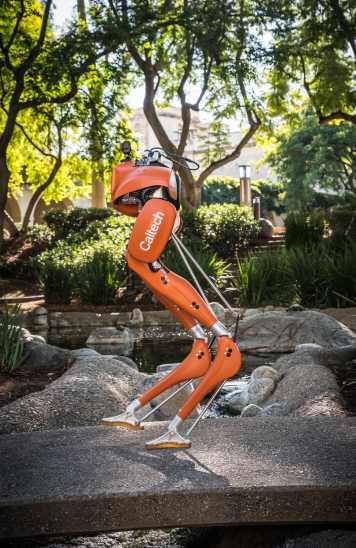
Seminar: Automatic Control of Autonomous Robots
Abstract:
Science fiction has long promised a world of robotic possibilities: from humanoid robots in the home, to wearable robotic devices that restore and augment human capabilities, to swarms of autonomous robotic systems forming the backbone of the cities of the future, to robots enabling exploration of the cosmos. With the goal of ultimately achieving these capabilities on robotic systems, this talk will present a unified optimization-based control framework for realizing dynamic behaviors in an efficient, provably correct and safety-critical fashion. The application of these ideas will be demonstrated experimentally on a wide variety of robotic systems, including swarms of rolling and flying robots with guaranteed collision-free behavior, bipedal and humanoid robots capable of achieving dynamic walking and running behaviors that display the hallmarks of natural human locomotion, and robotic assistive devices aimed at restoring mobility. The ideas presented will be framed in the broader context of seeking autonomy on robotic systems with the goal of getting robots into the real-world - a vision centered on combining able robotic bodies with intelligent artificial minds.
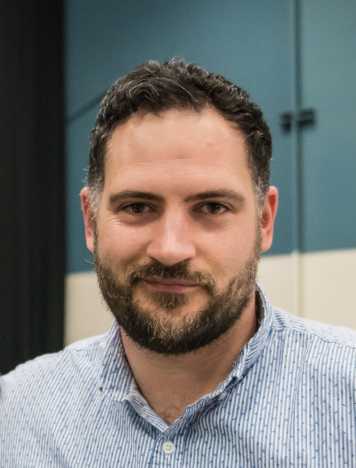
Biography:
Aaron D. Ames is the Bren Professor of Mechanical and Civil Engineering and Control and Dynamical Systems at the California Institute of Technology. Prior to joining Caltech, he was an Associate Professor in Mechanical Engineering and Electrical & Computer Engineering at the Georgia Institute of Technology. Dr. Ames received a B.S. in Mechanical Engineering and a B.A. in Mathematics from the University of St. Thomas in 2001, and he received a M.A. in Mathematics and a Ph.D. in Electrical Engineering and Computer Sciences from UC Berkeley in 2006. He served as a Postdoctoral Scholar in Control and Dynamical Systems at Caltech from 2006 to 2008, and began is faculty career at Texas A&M University in 2008. At UC Berkeley, he was the recipient of the 2005 Leon O. Chua Award for achievement in nonlinear science and the 2006 Bernard Friedman Memorial Prize in Applied Mathematics. Dr. Ames received the NSF CAREER award in 2010, and is the recipient of the 2015 Donald P. Eckman Award recognizing an outstanding young engineer in the field of automatic control. His research interests span the areas of robotics, nonlinear control and hybrid systems, with a special focus on applications to bipedal robotic walking—both formally and through experimental validation. His lab designs, builds and tests novel bipedal robots, humanoids and prostheses with the goal of achieving human-like bipedal robotic locomotion and translating these capabilities to robotic assistive devices. The application of these ideas range from increased autonomy in robots to improving the locomotion capabilities of the mobility impaired.
Seminar: Event-driven and Data-driven Control and Optimization in Cyber-physical Systems
Abstract:
This presentation will focus on two new major directions in the evolution of systems and control theory. The first is the emergence of the event-driven paradigm as an alternative viewpoint, complementary to the time-driven approach, for modeling, sampling, estimation, control, and optimization of dynamic systems that can provide dramatic energy savings in networked settings among other advantages. The second is the ubiquitous availability of data for both off-line and on-line processing. This opens up new opportunities for data-driven control and optimization methods that combine analytical system models with data. We will describe a general framework for event-driven and data-driven optimization that applies to Cyber-Physical Systems (CPS) with applications to cooperative multi-agent systems and to "smart cities".
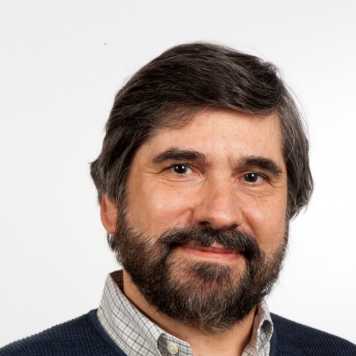
Biography:
Christos G. Cassandras is Distinguished Professor of Engineering at Boston University. He is Head of the Division of Systems Engineering, Professor of Electrical and Computer Engineering, and co-founder of Boston University’s Center for Information and Systems Engineering (CISE). He received degrees from Yale University (B.S., 1977), Stanford University (M.S.E.E., 1978), and Harvard University (S.M., 1979; Ph.D., 1982). In 1982-84 he was with ITP Boston, Inc. where he worked on the design of automated manufacturing systems. In 1984-1996 he was a faculty member at the Department of Electrical and Computer Engineering, University of Massachusetts/Amherst. He specializes in the areas of discrete event and hybrid systems, cooperative control, stochastic optimization, and computer simulation, with applications to computer and sensor networks, manufacturing systems, and transportation systems. He has published over 400 refereed papers in these areas, and six books. He has guest-edited several technical journal issues and serves on several journal Editorial Boards. In addition to his academic activities, he has worked extensively with industrial organizations on various systems integration projects and the development of decision-support software. He has most recently collaborated with The MathWorks, Inc. in the development of the discrete event and hybrid system simulator SimEvents.
Dr. Cassandras was Editor-in-Chief of the IEEE Transactions on Automatic Control from 1998 through 2009 and has also served as Editor for Technical Notes and Correspondence and Associate Editor. He is currently an Editor of Automatica. He was the 2012 President of the IEEE Control Systems Society (CSS). He has also served as Vice President for Publications and on the Board of Governors of the CSS, as well as on several IEEE committees, and has chaired several conferences. He has been a plenary/keynote speaker at numerous international conferences, including the American Control Conference in 2001, the IEEE Conference on Decision and Control in 2002 and
2016, and the 20th IFAC World Congress in 2017 and has also been an IEEE Distinguished Lecturer.
He is the recipient of several awards, including the 2011 IEEE Control Systems Technology Award, the Distinguished Member Award of the IEEE Control Systems Society (2006), the 1999 Harold Chestnut Prize (IFAC Best Control Engineering Textbook) for Discrete Event Systems: Modeling and Performance Analysis, a 2011 prize and a 2014 prize for the IBM/IEEE Smarter Planet Challenge competition (for a "Smart Parking" system and for the analytical engine of the Street Bump system respectively), the 2014 Engineering Distinguished Scholar Award at Boston University, several honorary professorships, a 1991 Lilly Fellowship and a 2012 Kern Fellowship. He is a member of Phi Beta Kappa and Tau Beta Pi. He is also a Fellow of the IEEE and a Fellow of the IFAC.
Seminar: Least Squares Optimal Identification of LTI SISO Dynamical Models is an Eigenvalue Problem
Abstract:
The last 50 years, we have witnessed tremendous progress in model-based control design and applications. Both the solution to the steady state LQR problem and the Kalman filter, ultimately reduce to an eigenvalue problem (which is hidden in the matrix Riccati equations involved). The same observation applies to the H-infty framework, with different Riccati equations.
But what about the models? Are they optimal in any sense, as often they are obtained from some non-linear optimization method? We will show how least squares optimal models for LTI SISO systems, derive from an eigenvalue problem, hence achieving an important landmark on optimal identification for this type of models. We focus on LTI SISO models, with at most one observed input and/or output data record, and at most one "unobserved" (typically assumed to be white) noise input (called "latency"). In the most general case, also the inputs and outputs are corrupted by additive measurement noise (called ‘misfit’). This class of models covers a lot of special cases, like AR, MA, ARMA, ARMAX, OE (Output-Error), Box-Jenkins, EIV (Errors-in-Variables), dynamic total least squares, and also includes models that up to now have not been described in the literature.
The results we will elaborate on, are the following:
- Firstly, we show that, using Lagrange multipliers, the least squares optimal model parameters, and the estimated latency and noise sequences, constitute a stationary point of the derivatives of the Lagrangean, which is equivalent to a (potentially large) set of multivariate polynomials. One (or some) of these stationary points provide the global minimum.
- We show that all stationary points of such multivariate polynomial optimization problems, correspond to the eigenvalues of a multi-dimensional (possibly singular) autonomous (nD-)shift invariant dynamical system.
- These eigenvalues can be obtained numerically by applying nD-realization theory in the null space calculated from a so-called Macaulay matrix. This matrix is built from the data, is a highly structured, quasi-Toeplitz matrix and in addition sparse. Revealing the number of stationary points that are finite, their structure and the solutions at infinity, requires a series of rank tests (SVDs) and eigenvalue calculations.
- We show how the parameters of the models are the eigenvalues of a multi-valued eigenvalue problem, and the estimated misfit and latency sequences are (parts) of corresponding eigenvectors. The implication is a serious reduction in computational complexity.
- Finally, we show how also this multi-valued eigenvalue problem ultimately reduces to an ‘ordinary’ (generalized) eigenvalue problem (i.e. in one variable, which is e.g. the minimal value of the least squares objective function).
Our results put many identification methods that have been described in the signal processing and identification literature, in a new perspective (think of VARPRO, IQML, "noisy" realization, PEM (Prediction-Error-Methods), Riemannian SVD, ….): all of them generate (often very good) "heuristic" algorithms, but here for the first time, we will show how all of them in fact are heuristic attempts to find the minimal eigenvalue of a large matrix.
The algorithms we come up with are guaranteed to find the global minima, using the well understood machinery of eigenvalue solvers and singular value decomposition algorithms. We will elaborate on the computational challenges (e.g. the fact that we only need to compute one eigenvalue-eigenvector pair of a large matrix, namely the one corresponding to the global optimum).
We will also elaborate on some preliminary system theoretic interpretations of these results (e.g. that misfit and latency error sequences are also highly structured in a system theoretic sense), and on some ideas on how to incorporate a priori information (e.g. power spectra) into these methods.
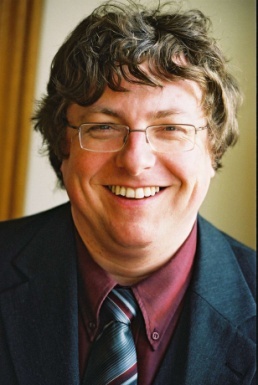
Biography:
Bart De Moor was born Tuesday July 12, 1960 in Halle, Belgium. He is married and has three children. He obtained his Master Degree in Electrical Engineering in 1983 and a PhD in Engineering in 1988 at the KU Leuven. For 2 years, he was a Visiting Research Associate at Stanford University (1988-1990) at the departments of EE (ISL, Prof. Kailath) and CS (Prof. Golub). Currently, he is a full professor at the Department of Electrical Engineering in the research group STADIUS, and a guest professor at the University of Siena.
His research interests are in numerical linear algebra, algebraic geometry and optimization, system theory and system identification, quantum information theory, control theory, datamining, information retrieval and bio-informatics (see publications on external page http://www.bartdemoor.be). He is or has been the coordinator of numerous research projects and networks funded by regional, federal and European funding agencies. He ranks 17th in the top H-index for Computer Science worldwide and 3rd in Europe.
Currently, he is leading a research group of 10 PhD students and 4 postdocs and in the recent past, 82 PhDs were obtained under his guidance. He has been teaching at several universities in Europe and the US. He is/was a member of several scientific and professional organizations, jury member of several scientific and industrial awards, and chairman or member of international conferences and educational and scientific review and selection committees, including the European Research Council. He is/was an associate editor of and reviewer for several scientific journals. His work has won him several scientific awards (Leybold-Heraeus Prize (1986), Leslie Fox Prize (1989), Guillemin-Cauer best paper Award of the IEEE Transactions on Circuits and Systems (1990), Laureate of the Belgian Royal Academy of Sciences (1992), bi-annual Siemens Award (1994), best paper award of Automatica (1996), IEEE Signal Processing Society Best Paper Award (1999)). In November 2010, he received the 5-annual FWO Excellence Award out of the hands of King Albert II of Belgium. Since 2004, he is a fellow of the IEEE and since 2017 a SIAM Fellow. Since 2000 he has been member of the Royal Academy of Belgium for Science and Arts.
From 1991-1999 he was the Head of Cabinet and/or Main Advisor on Science and Technology of several ministers of the Belgian Federal Government (Demeester, Martens) and the Flanders Regional Governments (Demeester, Van den Brande). From December 2005 to July 2007, he was the Head of Cabinet on socio-economic policy of the minister-president of Flanders, Yves Leterme, capacity in which he was the coordinator of the socio-economic business plan for the Flemish region.
He co-founded 7 spinoff companies, 6 of which are still active. He was or is in the Board of the Flemish Interuniversity Institute for Biotechnology, the Study Center for Nuclear Energy, the Institute for Broad Band Technology, the Flemish Children Science Center Technopolis, the Alamire Foundation, the AstraZeneca Foundation and several other scientific and cultural organizations. He is co-founder of the philosophical think tank Worldviews. In 2016 he founded the Health Tech Experience Center Health House.
He was the Chairman of the Industrial Research Fund of the KU Leuven, Chairman of the Hercules Foundation (scientific infrastructure funding) and is member of the Board of the Danish National Research Foundation. As a vice-rector for International Policy (2009-2013), he was a member of the Executive Committee and the Academic Council of the KU Leuven and of the Board of Directors of the Association KU Leuven. He also was the Chairman of the Committee on Internationalisation and Development Cooperation of the VLUHR. He is a co-founder and was Board member of the International School of Leuven. Since 2018 hes has also been member of ESGAB nominated by the European Council.
He made regular television appearances in the Science Show "Hoe?Zo!" on national television in Belgium, had a regular science talk on Radio 2 and patrons the "Flemish Youth Technology Olympiade".
Seminar: Brain-inspired non-von Neumann Computing
Abstract:
In today’s computing systems based on the conventional von Neumann architecture, there are distinct memory and processing units. Performing computations results in a significant amount of data being moved back and forth between the physically separated memory and processing units. This costs time and energy, and constitutes an inherent performance bottleneck. This has triggered research efforts to unravel and understand the highly efficient computational paradigm of the human brain, with the aim of creating brain-inspired computing systems. IBM with its fully digital TrueNorth chip architecture reached a key milestone in mimicking neural networks “in silico”. Besides fully digital approaches, also analog and hybrid architectures are being investigated.
Most recently, post-silicon nanoelectronic devices with memristive properties are also finding applications beyond the realm of memory. It is becoming increasingly clear that for application areas such as cognitive computing, we need to transition to computing architectures in which memory and logic coexist in some form. Brain-inspired neuromorphic computing and the fascinating new area of in-memory computing or computational memory are two key non-von Neumann approaches being researched. A critical requirement in these novel computing paradigms is a very-high-density, low-power, variable-state, programmable and non-volatile nanoscale memory device. Phase-change-memory (PCM) devices based on chalcogenide phase-change materials, such as Ge2Sb2Te5, are well suited to address this need owing to their multi-level storage capability and potential scalability.
Spiking neural networks (SNNs) are considered by many as the third-generation neural networks. It is widely believed that SNNs are computationally more powerful because of the added temporal dimension. PCM devices could emulate neuronal and synaptic dynamics. Such phase-change neurons also exhibit an intrinsic stochasticity and can thus be used for the representation of high-frequency signals via population coding. In general, such phase-change neurons and synapses can be used to realize SNNs and associated learning rules in a highly efficient manner. I will present some recent theoretical results and experimental demonstrations of large-scale spiking neural networks realized using PCM-based neurons and synapses.
In-memory computing is another brain-inspired non-Neumann approach. In in-memory computing, the physics of nanoscale memory devices as well as the organization of PCM devices in crossbar arrays are exploited to perform certain computational tasks within the memory unit. I will present large-scale experimental demonstrations using about one million PCM devices organized to perform high-level computational primitives, such as compressed sensing, linear solvers and temporal correlation detection. Moreover, I will discuss the efficacy of this approach to efficiently address the training and inference of deep neural networks. The results show that this co-existence of computation and storage at the nanometer scale could be the enabler for new, ultra-dense, low-power, and massively parallel computing systems.
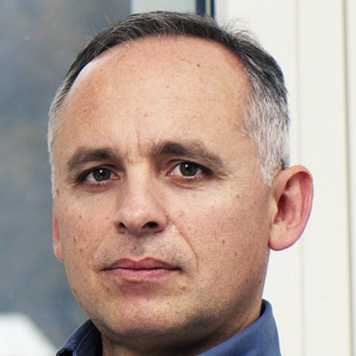
Biography:
Evangelos Eleftheriou received a B.S degree in Electrical Engineering from the University of Patras, Greece, in 1979, and M.Eng. and Ph.D. degrees in Electrical Engineering from Carleton University, Ottawa, Canada, in 1981 and 1985, respectively. In 1986, he joined the IBM Research – Zurich Laboratory in Rüschlikon, Switzerland, as a Research Staff Member. After serving as head of the Cloud and Computing Infrastructure department of IBM Research – Zurich for many years, Dr. Eleftheriou returned to a research position in 2018 to strengthen his focus on neuromorphic computing and to coordinate the Zurich Lab's activities with those of the global Research efforts in this field.
His research interests focus on enterprise solid-state storage, storage for big data, neuromorphic computing, and non-von Neumann computing architecture and technologies in general. He has authored or coauthored about 200 publications, and holds over 160 patents (granted and pending applications).
In 2002, he became a Fellow of the IEEE. He was co-recipient of the 2003 IEEE Communications Society Leonard G. Abraham Prize Paper Award. He was also co-recipient of the 2005 Technology Award of the Eduard Rhein Foundation. In 2005, he was appointed IBM Fellow for his pioneering work in recording and communications techniques, which established new standards of performance in hard disk drive technology. In the same year, he was also inducted into the IBM Academy of Technology. In 2009, he was co-recipient of the IEEE CSS Control Systems Technology Award and of the IEEE Transactions on Control Systems Technology Outstanding Paper Award. In 2016, he received an honoris causa professorship from the University of Patras, Greece.
In 2018, he was inducted as a foreign member into the National Academy of Engineering for his contributions to digital storage and nanopositioning technologies, as implemented in hard disk, tape, and phase-change memory storage systems.
Seminar: Temporal Logics for Multi-Agent Systems
Abstract:
Temporal logic formalizes reasoning about the possible behaviors of a system over time. For example, a temporal formula may stipulate that an occurrence of event A may be, or must be, followed by an occurrence of event B. Traditional temporal logics, however, are insufficient for reasoning about multi-agent systems such as control systems. In order to stipulate, for example, that a controller can ensure that A is followed by B, references to agents, their capabilities, and their intentions must be added to the logic, yielding Alternating-time Temporal Logic (ATL). ATL is a temporal logic that is interpreted over multi-player games, whose players correspond to agents that pursue temporal objectives. The hardness of the model-checking problem -whether a given formula is true for a given multi-agent system- depends on how the players decide on the next move in the game (for example, whether they take turns, or make independent concurrent decisions, or bid for the next move), how the outcome of a move is computed (deterministically or stochastically), how much memory the players have available for making decisions, and which kind of objectives they pursue (qualitative or quantitative). The expressiveness of ATL is still insufficient for reasoning about equilibria and related phenomena in non-zero-sum games, where the players may have interfering but not necessarily complementary objectives. For this purpose, the behavioral strategies (a.k.a. policies) of individual players can been added to the logic as quantifiable first-order entities, yielding Strategy Logic. We survey several known results about these multi-player game logics and point out some open problems.
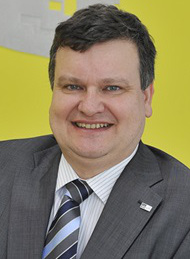
Biography:
Tom Henzinger is president of IST Austria (Institute of Science and Technology Austria). He holds a PhD degree from Stanford University (1991), and honorary doctorates from Fourier University in Grenoble and from Masaryk University in Brno. He was professor at Cornell University (1992-95), the University of California, Berkeley (1996-2004), and EPFL (2004-09). He was also director at the Max-Planck Institute for Computer Science in Saarbruecken. His research focuses on modern systems theory, especially models, algorithms, and tools for the design and verification of reliable software, hardware, and embedded systems. His HyTech tool was the first model checker for mixed discrete-continuous systems. He is an ISI highly cited researcher, a member of Academia Europaea and of the German and Austrian Academies of Sciences, and a fellow of the AAAS, ACM, and IEEE. He received the Milner Award of the Royal Society, the Wittgenstein Award of the Austrian Science Fund, and an Advanced Investigator Grant of the European Research Council.
Seminar: Understanding Acceleration and Escape from Saddle Points in first-order Optimization
Abstract:
In the first part of the talk, I focus on studying gradient-based optimization methods obtained by directly discretizing a second-order ordinary differential equation (ODE) corresponding to gradient dynamics with mass and friction. When the function is smooth enough, we show that acceleration can be achieved by a stable discretization of this ODE using standard Runge-Kutta integrators. Specifically, we show under Lipschitz-gradient and convexity the sequence of iterates generated by discretizing the proposed second-order ODE achieves acceleration, without any need for specialized integration schemes.
Furthermore, we introduce a new local flatness condition on the objective, under which rates even faster than O(1/N^2) can be achieved with low-order integrators and only gradient information. Notably, this flatness condition is satisfied by several standard loss functions used in machine learning.
In the second part of the talk, I focus on escaping from saddle points in smooth nonconvex optimization problems subject to a convex constraint. We propose a generic framework that yields convergence to a second-order stationary point (SOSP) of the problem if the constraint set $C$ is simple for a quadratic objective function. To be more precise, our results hold if one can solve a quadratic program over the set $C$ up to a constant factor. Under this condition, we show that the sequence of iterates generated by the proposed method reaches an SOSP in poly-time. We further characterize the overall arithmetic operations to reach an SOSP when the convex set $C$ can be written as a set of quadratic constraints. Finally, we extend our results to the stochastic setting and characterize the number of stochastic gradient and Hessian evaluations required to reach an SOSP.
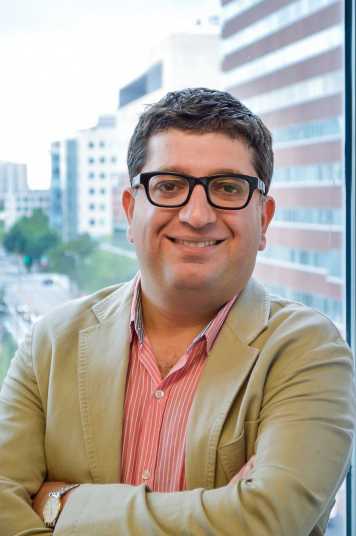
Biography:
Ali Jadbabaie is the JR East Professor of Engineering and Associate Director of the Institute for Data, Systems, and Society at MIT, where he is also on the faculty of the Department of Civil and Environmental Engineering and a principal investigator in the Laboratory for Information and Decision Systems (LIDS), and the director of the Sociotechnical Systems Research Center, one of MIT's 13 research laboratories. He received his Bachelors (with high honors) from Sharif University of Technology in Tehran, Iran, a Masters degree in electrical and computer engineering from the University of New Mexico, and his PhD in control and dynamical systems from the California Institute of Technology. He was a postdoctoral scholar at Yale University before joining the faculty at Penn in July 2002 where he was the Alfred Fitler Moore a Professor of Network Science. He was the inaugural editor-in-chief of IEEE Transactions on Network Science and Engineering, a new interdisciplinary journal sponsored by several IEEE societies. He is a recipient of a National Science Foundation Career Award, an Office of Naval Research Young Investigator Award, the O. Hugo Schuck Best Paper Award from the American Automatic Control Council, and the George S. Axelby Best Paper Award from the IEEE Control Systems Society. His students have been winners and finalists of student best paper awards at various ACC and CDC conferences. He is an IEEE fellow and a recipient of the 2016 Vannevar Bush Fellowship from the office of Secretary of Defense, and a member of the National Academies of Science, Engineering, and Medicine's Intelligence Science and Technology Expert Group (ISTEG). His current research interests are in distributed decision making and optimization, multi-agent coordination and control, network science, and network economics.
Seminar: Reinforcement Learning for Unknown Autonomous Systems
Abstract:
System Identification followed by control synthesis has long been the dominant paradigm for control engineers. And yet, many autonomous systems must learn and control at the same time. Adaptive Control Theory has indeed been motivated by this need. But it has focused on asymptotic stability while many contemporary applications demand finite time (non-asymptotic) performance optimality. Results in stochastic adaptive control theory are sparse with any such algorithms being impractical for real-world implementation. We propose Reinforcement Learning algorithms inspired by recent developments in Online (bandit) Learning.
Two settings will be considered: Markov decision processes (MDPs) and Linear stochastic systems. We will introduce a posterior-sampling based regret-minimization learning algorithm that optimally trades off exploration v. exploitation and achieves order optimal regret. This is a practical algorithm that obviates the need for expensive computation and achieves non-asymptotic regret optimality. I will then talk about a general non-parametric stochastic system model on continuous state spaces. Designing universal control algorithms (that work for any problem) for such settings (even with known model) that are provably (approximately) optimal has long been a very challenging problem in both Stochastic Control and Reinforcement Learning. I will propose a simple algorithm that combines randomized function approximation in universal function approximation spaces with Empirical Q-Value Learning which is not only universal but also approximately optimal with high probability. In closing, I would like to argue that the problems of Reinforcement Learning present a new opportunity for Controls and some interesting future directions.
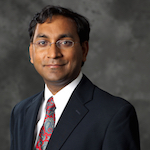
Biography:
Rahul Jain is the K. C. Dahlberg Early Career Chair and Associate Professor of Electrical Engineering, Computer Science* and ISE* (*by courtesy) at the University of Southern California. He received a B.Tech from the IIT Kanpur, and an MA in Statistics and a PhD in EECS from the University of California, Berkeley. He has been a recipient of the NSF CAREER award, the Office of Naval Research Young Investigator award, an IBM Faculty award, the James H. Zumberge Faculty Research and Innovation Award, and is currently a US Fulbright Scholar. His interests span reinforcement learning, stochastic control, stochastic networks, and game theory, and power systems and healthcare on the applications side.
Seminar: Fastest Convergence for Reinforcement Learning
Abstract:
There are two well known Stochastic Approximation techniques that are known to have optimal rate of convergence (measured in terms of asymptotic variance): the Stochastic Newton-Raphson (SNR) algorithm [a matrix gain algorithm that resembles the deterministic Newton-Raphson method], and the Ruppert-Polyak averaging technique. This talk will present new applications of these concepts for reinforcement learning.
1. Introducing Zap Q-Learning. In recent work, first presented at NIPS 2017, it is shown that a new formulation of SNR provides a new approach to Q-learning that has provably optimal rate of convergence under general assumptions, and astonishingly quick convergence in numerical examples. In particular, the standard Q-learning algorithm of Watkins typically has infinite asymptotic covariance, and in simulations the Zap Q-Learning algorithm exhibits much faster convergence than the Ruppert-Polyak averaging method. The only difficulty is the matrix inversion required in the SNR recursion.
2. A remedy is proposed based on a variant of Polyak’s heavy-ball method. For a special choice of the “momentum" gain sequence, it is shown that the parameter estimates obtained from the algorithm are essentially identical to those obtained using SNR. This new algorithm does not require matrix inversion. In simulations it is found that the sample paths of the two algorithms couple. A theoretical explanation for coupling is established for linear recursions.
Along with these new results, the talk will survey and unify popular approaches to deterministic optimization that form the foundation of this work.
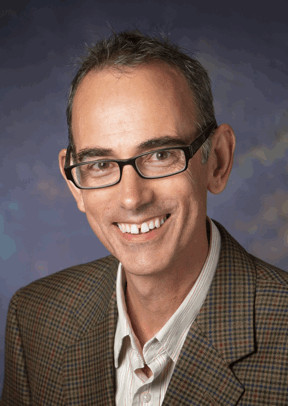
Biography:
Sean Meyn received the BA degree in mathematics from the University of California, Los Angeles, in 1982 and the PhD degree in electrical engineering from McGill University, Canada, in 1987 (with Prof. P. Caines). He is now Professor and Robert C. Pittman Eminent Scholar Chair in the Department of Electrical and Computer Engineering at the University of Florida, the director of the Laboratory for Cognition and Control, and director of the Florida Institute for Sustainable Energy. His academic research interests include theory and applications of decision and control, stochastic processes, and optimization. He has received many awards for his research on these topics, and is a fellow of the IEEE. He has held visiting positions at universities all over the world, including the Indian Institute of Science, Bangalore during 1997-1998 where he was a Fulbright Research Scholar. During his latest sabbatical during the 2006-2007 academic year he was a visiting professor at MIT and United Technologies Research Center (UTRC). His award-winning 1993 monograph with Richard Tweedie, Markov Chains and Stochastic Stability, has been cited thousands of times in journals from a range of fields. The latest version is published in the Cambridge Mathematical Library. For the past ten years his applied research has focused on engineering, markets, and policy in energy systems. He regularly engages in industry, government, and academic panels on these topics, and hosts an annual workshop at the University of Florida.
Seminar: Exploiting Sparsity in Semidefinite and Sum of Squares Programming
Abstract:
Semidefinite and sum of squares optimization have found a wide range of applications, including control theory, fluid dynamics, machine learning, and power systems. In theory they can be solved in polynomial time using interior-point methods. However, these methods are only practical for small- to medium- sized problem instances.
For large instances, it is essential to exploit or even impose sparsity and structure within the problem in order to solve the associated programs efficiently. In this talk I will present recent results on the analysis and design of networked systems, where chordal sparsity can be used to decompose the resulting SDPs, and solve an equivalent set of smaller semidefinite constraints. I will also discuss how sparsity and operator-splitting methods can be used to speed up computation of large SDPs and introduce our open-source solver CDCS. Lastly, I will extend the decomposition result on SDPs to SOS optimization with polynomial constraints, revealing a practical way to connect SOS optimization and DSOS/SDSOS optimization for sparse problem instances.
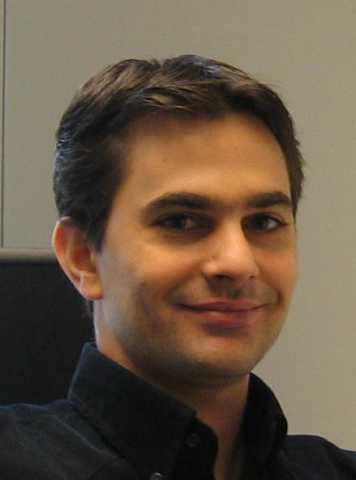
Biography:
Antonis Papachristodoulou joined the University of Oxford in 2006, where he is currently Professor of Engineering Science and a Tutorial Fellow in Worcester College, Oxford. Since 2015, he has been EPSRC Fellow and Director of the EPSRC & BBSRC Centre for Doctoral training in Synthetic Biology. He holds an MA/MEng in Electrical and Information Sciences from the University of Cambridge (2000) and a PhD in Control and Dynamical Systems from the California Institute of Technology, with a PhD Minor in Aeronautics (2005). In 2015 he was awarded the European Control Award for his contributions to robustness analysis and applications to networked control systems and systems biology and the O. Hugo Schuck Best Paper Award. He serves regularly on Technical Programme Committees for conferences, and was associate editor for Automatica and IEEE Transactions on Automatic Control.
Seminar: Multi-agent distributed optimization over networks and its application to energy systems
Abstract:
The well-functioning of our modern society rests on the reliable and uninterrupted operation of large scale complex infrastructures, which are more and more exhibiting a network structure with a high number of interacting components/agents. Energy and transportation systems, communication and social networks are a few, yet prominent, examples of such large scale multi-agent networked systems. Depending on the specific case, agents may act cooperatively to optimize the overall system performance or compete for shared resources. Based on the underlying communication architecture, and the presence or not of a central regulation authority, either decentralized
or distributed decision making paradigms are adopted.
In this talk, we address the interacting and distributed nature of cooperative multi-agent systems arising in the energy application domain. More specifically, we present our recent results on the development of a unifying distributed optimization framework to cope with the main complexity features that are prominent in such systems, i.e.: heterogeneity, as we allow the agents to have different objectives and physical/technological constraints; privacy, as we do not require agents to disclose their local information; uncertainty, as we take into account uncertainty affecting the agents locally and/or globally; and combinatorial complexity, as we address the case of discrete decision variables.
This is a joint work with Alessandro Falsone, Simone Garatti, and Kostas Margellos.
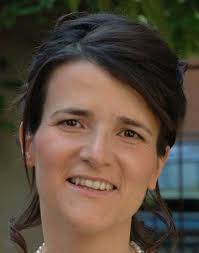
Biography:
Maria Prandini received her laurea degree in Electrical Engineering (summa cum laude) from Politecnico di Milano (1994) and her Ph.D. degree in Information Technology from Università di Brescia (1998). She was a postdoctoral researcher at the University of California at Berkeley (1998-2000). She also held visiting positions at Delft University of Technology (1998), Cambridge University (2000), University of California at Berkeley (2005), and Swiss Federal Institute of Technology Zurich (2006). In 2002, she became an assistant professor in systems and control at Politecnico di Milano, where she is currently a full professor.
She has been in the program committees of several international conferences, co-chair of HSCC 2018, and she has been appointed program chair of IEEE CDC 2021. She serves as an associate editor for IEEE Transactions on Network Systems, and previously for IEEE Transactions on Automatic Control, IEEE Transactions on Control Systems Technology, and Nonlinear Analysis: Hybrid Systems. She is member of IFAC Technical Committee on Discrete Event and Hybrid Systems since 2008 and of the IFAC Policy Committee for the triennium 2017-20. She was editor for the CSS Electronic Publications (2013-15), elected member of the IEEE CSS Board of Governors (2015-17), and IEEE CSS Vice-President for Conference Activities (2016-17). She is senior member of the IEEE.
Her research interests include stochastic hybrid systems, randomized algorithms, distributed and data-based optimization, multi-agent systems, and the application of control theory to transportation and energy systems.
Seminar: Time Series As A Matrix
Abstract:
We consider the task of interpolating and forecasting a time series in the presence of noise and missing data. As the main contribution of this work, we introduce an algorithm that transforms the observed time series into a matrix, utilizes matrix estimation as a black-box to simultaneously recover missing values and de-noise observed entries, and performs linear regression to make predictions. We argue that this method provides meaningful imputation and forecasting for a large class of models: finite sum of harmonics (which approximate stationary processes), non-stationary sub-linear trends, Linear-Time-Invariant (LTI) systems, and their additive mixtures. In general, our algorithm recovers the hidden state of dynamics based on its noisy observations, like that of a Hidden Markov Model (HMM), provided the dynamics obey the above stated models. As an important application, it provides a robust algorithm for "synthetic control'' for causal inference.
This is based on joint works with Anish Agarwal, Muhammad Amjad and Dennis Shen (all at MIT).
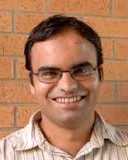
Biography:
Devavrat Shah is a Professor with the department of Electrical Engineering and Computer Science at Massachusetts Institute of Technology. His current research interests are at the interface of Statistical Inference and Social Data Processing. His work has been recognized through prize paper awards in Machine Learning, Operations Research and Computer Science, as well as career prizes including 2010 Erlang prize from the INFORMS Applied Probability Society and 2008 ACM Sigmetrics Rising Star Award. He is a distinguished young alumni of his alma mater IIT Bombay and Adjunct Professor at Tata Institute of Fundamental Research, Mumbai. He founded the machine learning start-up Celect, Inc. which helps retailer with optimizing inventory by accurate demand forecasting.
Seminar: The Information Knot Tying Sensing and Control and the Emergence Theory of Deep Representation Learning
Abstract:
Internal representations of the physical environment, inferred from sensory data, are believed to be crucial for interaction with it, but until recently lacked sound theoretical foundations. Indeed, some of the practices for high-dimensional sensor streams like imagery seemed to contravene basic principles of Information Theory: Are there non-trivial functions of past data that ‘summarize’ the ‘information’ it contains that is relevant to decision and control tasks? What ‘state’ of the world should an autonomous system maintain? How would such a state be inferred? What properties should it have? Is there some kind of ‘separation principle’, whereby a statistic (the state) of all past data is sufficient for control and decision tasks? I will start from defining an optimal representation as a (stochastic) function of past data that is sufficient (as good as the data) for a given task, has minimal (information) complexity, and is invariant to nuisance factors affecting the data but irrelevant for a task. Such minimal sufficient invariants, if computable, would be an ideal representation of the given data for the given task. I will then show that these criteria can be formalized into a variational optimization problem via the Information Bottleneck Lagrangian, and minimized with respect to a universal approximating class of function realized by deep neural networks. I will then specialize this program for control tasks, and show that it is possible to define and compute a ‘state’ that separates past data from future tasks, and has all the desirable properties that generalize the state of dynamical models customary in linear control systems, except for being highly non-linear and having high-dimension (in the millions).
References:
external page https://arxiv.org/pdf/1706.01350.pdf (JMLR 2018) and external page https://arxiv.org/abs/1710.11029 (ICLR 2018). external page https://www.annualreviews.org/doi/pdf/10.1146/annurev-control-060117-105140 (Annual Reviews 2018)
Joint work with Alessandro Achille and Pratik Chaudhari.
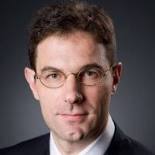
Biography:
Stefano Soatto is Professor of Computer Science and Electrical Engineering, and Director of the UCLA Vision Lab, in the Henry Samueli School of Engineering and Applied Sciences at UCLA. He is also Director of Applied Science at AWS/Amazon AI. He received his Ph.D. in Control and Dynamical Systems from the California Institute of Technology in 1996; he joined UCLA in 2000 after being Assistant and then Associate Professor of Electrical and Biomedical Engineering at Washington University, and Research Associate in Applied Sciences at Harvard University. Between 1995 and 1998 he was also Ricercatore in the Department of Mathematics and Computer Science at the University of Udine - Italy. He received his D.Ing. degree (highest honors) from the University of Padova- Italy in 1992. Dr. Soatto is the recipient of the David Marr Prize for work on Euclidean reconstruction and reprojection up to subgroups. He also received the Siemens Prize with the Outstanding Paper Award from the IEEE Computer Society for his work on optimal structure from motion. He received the National Science Foundation Career Award and the Okawa Foundation Grant. He was Associate Editor of the IEEE Transactions on Pattern Analysis and Machine Intelligence (PAMI) and a Member of the Editorial Board of the International Journal of Computer Vision (IJCV) and Foundations and Trends in Computer Graphics and Vision, Journal of Mathematical Imaging and Vision, SIAM Imaging. He is a Fellow of the IEEE.
Seminar: Approximate MPC for Feedback Control through Contact
Abstract:
For robots governed by smooth, controllable (possibly nonlinear) ODEs, linear MPC provides an extremely powerful tool for local stabilization of a fixed point or trajectory. The state of the art in multi-contact feedback control is much worse — we mostly rely on one-step lookahead (e.g. QP inverse dynamics) and or hand-designed state machines — the major missing piece is a systematic design procedure that can reason about stabilization “across contact modes”. As a result, legged robots struggle to recover from deviations from their pre-planned hybrid mode sequence, and there is an embarrassing paucity of feedback control theory to be found in the field of robot manipulation.
In this talk, I will argue that piecewise-affine (PWA) approximations of the system dynamics in floating-base coordinates are the natural and reasonable analog to linearization when locally stabilizing fixed-points or trajectories that lie on or near contact-mode boundaries. For these models, well-known results from explicit MPC tell us quite a bit about the feedback controller that optimizes a quadratic regulator objective (although it is not as nice as the affine case). The problem is primarily that the number of pieces quickly becomes too large for even moderately-sized problems. I will present a number of algorithms that we have been developing to efficiently compute the mixed-integer convex optimization problems that result from formulations like the piecewise-affine quadratic regulator (PWAQR), including some new tightness results leveraging disjunctive programming. I will also algorithms that approximate the explicit MPC solution, and discuss the prospects of actually having a turnkey solution that is anywhere near as powerful as LQR for smooth systems.
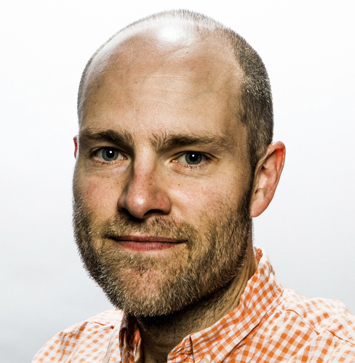
Biography:
Russ is the Toyota Professor of Electrical Engineering and Computer Science, Aeronautics and Astronautics, and Mechanical Engineering at MIT, the Director of the Center for Robotics at the Computer Science and Artificial Intelligence Lab, and the leader of Team MIT's entry in the DARPA Robotics Challenge. Russ is also the Vice President of Robotics Research at the Toyota Research Institute. He is a recipient of the NSF CAREER Award, the MIT Jerome Saltzer Award for undergraduate teaching, the DARPA Young Faculty Award in Mathematics, the 2012 Ruth and Joel Spira Teaching Award, and was named a Microsoft Research New Faculty Fellow.
Russ received his B.S.E. in Computer Engineering from the University of Michigan, Ann Arbor, in 1999, and his Ph.D. in Electrical Engineering and Computer Science from MIT in 2004, working with Sebastian Seung. After graduation, he joined the MIT Brain and Cognitive Sciences Department as a Postdoctoral Associate. During his education, he has also spent time at Microsoft, Microsoft Research, and the Santa Fe Institute.
Seminar: Cyber-physical Manufacturing Systems: Improving Productivity with Advanced Control
Abstract:
High-performance computing technologies, including high-speed networks, high-performance simulations, high-speed networks, and cloud computing, are revolutionizing manufacturing systems operations. Using existing hardware (robots, CNC machines, conveyors), but taking advantage of the vast amounts of data being collected in real-time, advanced control techniques can result in reduced downtime, improved quality, and overall improved productivity. This talk will describe how the integration of simulation and plant-floor data can enable new control approaches that are able to optimize the overall performance of manufacturing system operations. Both hierarchical and distributed control approaches will be discussed, and implementations on a testbed at the University of Michigan will be described. Collaborations with industry will be highlighted.
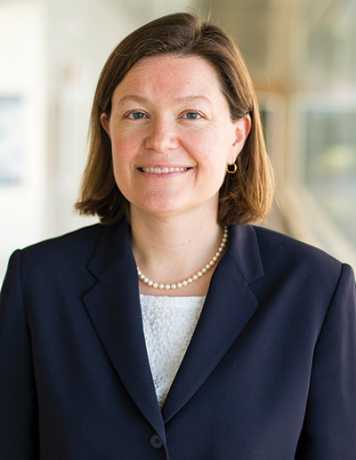
Biography:
Dawn M. Tilbury received the B.S. degree in Electrical Engineering, summa cum laude, from the University of Minnesota in 1989, and the M.S. and Ph.D. degrees in Electrical Engineering and Computer Sciences from the University of California, Berkeley, in 1992 and 1994, respectively. In 1995, she joined the Mechanical Engineering Department at the University of Michigan, Ann Arbor, where she is currently Professor, with a joint appointment as Professor of EECS. Her research interests include distributed control of mechanical systems with network communication, logic control of manufacturing systems, and trust in automated vehicles. She was elected Fellow of the IEEE in 2008 and Fellow of the ASME in 2012, and is a Life Member of SWE. Since June of 2017, she is on leave from the University of Michigan, serving as Assistant Director for Engineering at the US National Science Foundation (NSF).
Seminar: Global Optimality in Matrix Factorization, Tensor Factorization and Deep Learning
Abstract:
The past few years have seen a dramatic increase in the performance of recognition systems thanks to the introduction of deep networks for representation learning. However, the mathematical reasons for this success remain elusive. A key issue is that the neural network training problem is non-convex, hence optimization algorithms may not return a global minima. In addition, the regularization properties of algorithms such as dropout remain poorly understood. Building on ideas from convex relaxations of matrix factorizations, this work proposes a general framework which allows for the analysis of a wide range of non-convex factorization problems – including matrix factorization, tensor factorization, and deep neural network training. The talk will describe sufficient conditions under which a local minimum of the non-convex optimization problem is a global minimum and show that if the size of the factorized variables is large enough then from any initialization it is possible to find a global minimizer using a local descent algorithm. The talk will also present an analysis of the optimization and regularization properties of dropout in the case of matrix factorization.
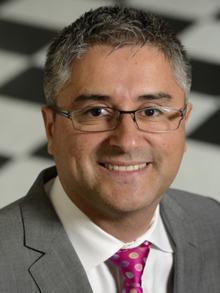
Biography:
Rene Vidal is the Herschel L. Seder Professor of Biomedical Engineering and the Inaugural Director of the Mathematical Institute for Data Science at The Johns Hopkins University. His research focuses on the development of theory and algorithms for the analysis of complex high-dimensional datasets such as images, videos, time-series and biomedical data. Dr. Vidal has been Associate Editor of TPAMI and CVIU, Program Chair of ICCV and CVPR, co-author of the book "Generalized Principal Component Analysis" (2016), and co-author of more than 200 articles in machine learning, computer vision, biomedical image analysis, hybrid systems, robotics and signal processing. He is a fellow of the IEEE, IAPR and Sloan Foundation, a ONR Young Investigator, and has received numerous awards for his work, including the 2012 J.K. Aggarwal Prize for "outstanding contributions to generalized principal component analysis (GPCA) and subspace clustering in computer vision and pattern recognition" as well as best paper awards in machine learning, computer vision, controls, and medical robotics.