Anesthesia
This research project is not currently active and this page is not regularly updated. It is provided here for archival purposes.
Over the past years our group has developed different model-based controllers for ventilation, blood pressure, depth of anesthesia and muscular relaxation (Former Projects). However, the major issue of controlling patient's analgesia is still open. Current research focuses on providing adequate analgesia during surgery, in the postoperative setting and for conscious sedation.
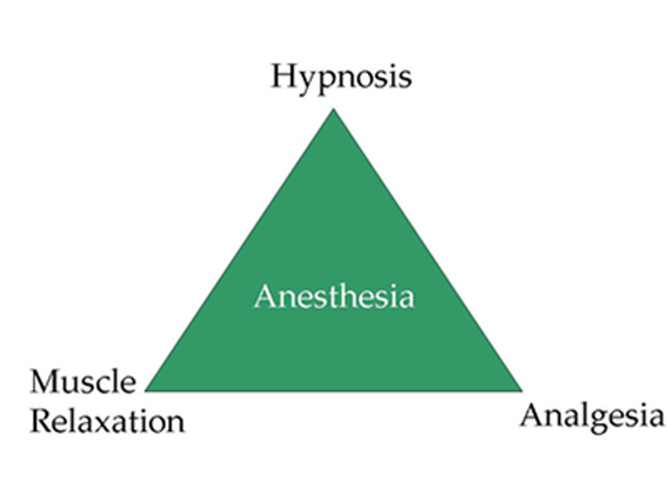
The first steps to introduce feedback control in anesthesia were undertaken more than ten years ago. The project encompasses all control system aspects, namely
- design of experiments and open loop identification
- modeling of biological complex systems
- statistical analysis of large population data
- control design and simulation
- pilot studies and clinical routine validation
Automated Control of Anesthesia was successfully tested during surgery on more than 200 patients and 650 hours.
The successful application of our ideas is made possible by the close cooperation with: the Department of Anaesthesiology at the external page University Hospital in Berne (Inselspital), where volunteers are enrolled for model identification and controllers are validated in clinical studies; external page Dräger, which enables us to use the most advanced technologies in anaesthesia; external page Sentec, which supports us with the latest noninvasive blood gas monitoring devices.
In April 2005, the Anesthesia Control Group, together with the partners in Bern and the University Hospital of Gent (Belgium), organized the workshop "Advanced Modeling and Control in Anesthesia", collecting 100 participants from all over the world. International experts on pharmacokinetic/pharmacodynamic modeling and control evaluated the current state of the art and developed a vision for future applications of such methods in clinical practice.
Adequate anesthesia can be defined as a reversible pharmacological state where the patient's muscle relaxation, analgesia and hypnosis are provided. Muscle relaxation is induced to facilitate the access to internal organs and to depress movement response to surgical stimulation. Analgesia is pain relief. Hypnosis is a general term indicating unconcsciousness and absence of post operative recall of events occurred during surgery. Anesthesiologists administer drugs and adjust several medical devices to achieve such goals and to compensate for the effect of surgical manipulation while maintainig the vital functons of the patient. This is done based on some vital parameter specific target values and monitor readings. Thus, anesthesiologists adopt the role of a feedback controller and it is natural to ask whether automatic controllers are capable of taking over and/or improving parts os such a complex decision process.
Over the past years our group has developed different model-based controllers for ventilation, blood pressure, depth of anesthesia and muscular relaxation (Former Projects). However, the major issue of controlling patient's analgesia is still open. Current research focuses on providing adequate analgesia during surgery, in the postoperative setting and for conscious sedation.
Adequate Analgesia During Surgery
Adequate Analgesia in the Post-operative Setting
The design of open loop (TCI pumps) and closed loop systems for the automatic delivery of the drugs are based on pharmacokinetic and pharmacodynamic models containing "population" parameters, i.e., representative of the "average" patient. These models are identified in ad-hoc studies, but it is known that the experimental setup influences the parameter estimation and, as a consequence, the performances of the model predictions. Therefore, one of our focuses is to define guidelines for optimal experiment design.
Optimal experiment design
Because of the large patients inter-variability, a model containing "population" parameters could fail to predict the response of a specific patient to a defined input. Therefore individualization of the model parameters is needed.
On-line Parameter Estimation
Adequate Analgesia During Surgery
The major challenge we are facing today for the control of analgesia during surgery is the lack of a specific sensor for the analgesic level of the unconscious patient. It even seems questionable to speak about pain perception when the subject is unconscious. However, even in the unconscious patient, pain activates a reaction, the so called stress response. Typical stress response reactions include for example pupil widening, increase of heart rate and blood pressure, contraction of skin vessels. Thus, one approach to the problem of measuring analgesia is to search for changes in the vital parameters of the patients, representing the body's response to the occurrence of a painful stimulus, that might be used as surrogate indicators of the experienced pain. For this, 20 volunteers were enrolled in a study designed to explore the effects of the hypnotic isoflurane and the analgesic alfentanil on several clinical end-points and to determine the reactions of such end-points to experimental painful stimuli.
Real-Time Estimation of Pain-Induced Mean Arterial Blood Pressure Variations
Mean Arterial Blood Pressure (MAP) is a patient's vital parameter and is constantly monitored during surgery or intensive care. Different events can lead to blood pressure changes during surgery. One of the most important ones is the stress response, the body's reaction to the painful stimulation induced by the surgeon. To contain complications and costs, non-invasive MAP measurement is usually preferred to any invasive measurement technique. The mostly diffused non-invasive device suffers from long sampling times, causing a critical loss of information of the fast pain-induced MAP variations. A robust and reliable estimation of blood pressure during the time span between two consecutive measurements would cover the lack of information and represent a major improvement providing the anesthetist continuous blood pressure information, while keeping stress for the patient, risks and costs minimal.
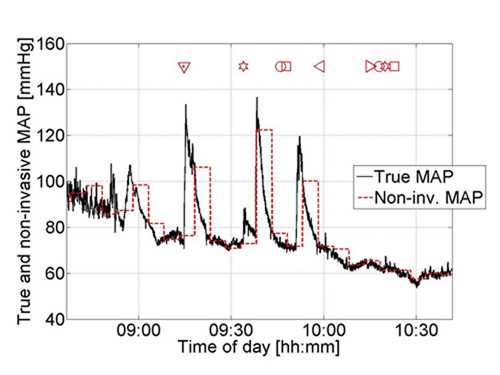
In the surgical setting, the anesthetist is able to obtain information about the general stress level of the patient by taking into account different signals simultaneously. Aim of this investigation is to try mimic the anesthetist by extracting information about the high frequency behavior of MAP from additional signals. On a set of 20 volunteers we investigated whether information about the variations in MAP during the time span between to consecutive non-invasive measurement could be derived from heart rate (HR). Combining this information, accounting for the fast MAP behavior, with the non-invasive measurement, accounting for the slow MAP behavior, MAP was continuously estimated. The method was proven to be accurate and robust concerning inter-patient variability. With this procedure, MAP can be estimated on-line without requiring additional sensors.
[Paper]
Influence of Pain on the Pulse Wave Amplitude - Statistical Analysis of Variance
According to the doctor's experience, the amplitude of the pulse wave signal (PWA) is expected to decrease after a painful stimulus following the contraction of the skin vessels induced by the stress response. Goal of this study is to analyze the pulse wave signal of volunteers under anaesthesia subject to painful stimulation in order to statistically assess the effect of pain on the signal amplitude and to determine wether the extend of the pain-induce signal variation is depends on the amount of drugs administered. The data from the volunteer study is analyzed by an analysis of variance (ANOVA).
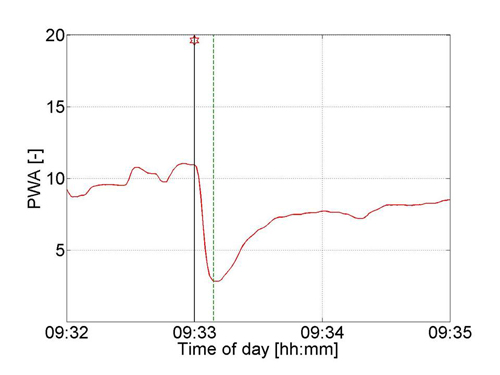
This method helps identify sources of variability among one or more potential sources The ANOVA has many similarities to a regression procedure. In this case, the dependent variable is only one, the pain-induced PWA variation, leading to an univariate analysis of variance, while the potential sources of variability are: the volunteer number, the stimulus number, the two drug concentrations and their interaction.
The performed analysis shows that pain induces a significant variation in the amplitude of the pulse wave signal. The extend of the pain-induced variation is highly dependent on the subject's sensitivity and is influenced by analgesic-hypnotic drug interactions and by fatigue. Both, drug interactions and fatigue tend to attenuate the body's response to the painful stimulation.
Adequate Analgesia in the Post-operative Setting
In the post-operative setting it is necessary to provide pain-relief from the invasive surgery. In this case, conversely to the surgical setting, the patient is conscious and the drug infusion rate can be adjusted according to the patient's pain level. For pain management drugs are usually combined because of the expected enhancement of the analgesic effect due to synergy. On the other hand, drug combination might lead to a parallel enhancement of the undesired effects. The major problem in investigating drug combinations is the number of possible combinations to be tested. Therefore, the challenging issue is how to determine in an efficient way the "optimal combination" leading to highest pain-relief with the lowest incidence of side effects. In general, there are two main approaches to optimization problems: the black-box or the modelling approach.
Black-box Optimization of Drug Combinations
Despite its great importance, the problem of optimizing therapeutic regimens has received very little formal attention in medicine. Berenmbaum (1990)developed a modified version of the simplex method, the partition method, able to deal with largely biased data such a patient data. The partition model represents the first application of a black box optimization method to the identification of optimal drug combinations. However, the partition method in its original formulation presents major drawback which limit their utility. We developed a novel optimization procedure and successfully applied it to the optimization of the combination of bupivacaine, fentanyl and clonidine.
[Paper]
The Well-being Model
Drugs are routinely combined in anesthesia and pain management to obtain an enhancement of the desired effects. However, a parallel enhancement of the undesired effects might take place as well, resulting in a limited therapeutic usefulness. Therefore, addressing the question of optimal drugs combinations, side effects must be taken into account. By extension of a previously published interaction model we propose a method to study drug interactions considering also their side effects. A general outcome parameter identified as "patient's well-being" is defined by superposition of positive and negative effects and response surfaces can be derived starting from the individual parameters of the drugs. The different effects of synergistics or antagonistics interactions on the response surface are consistent with clinical knowledge.
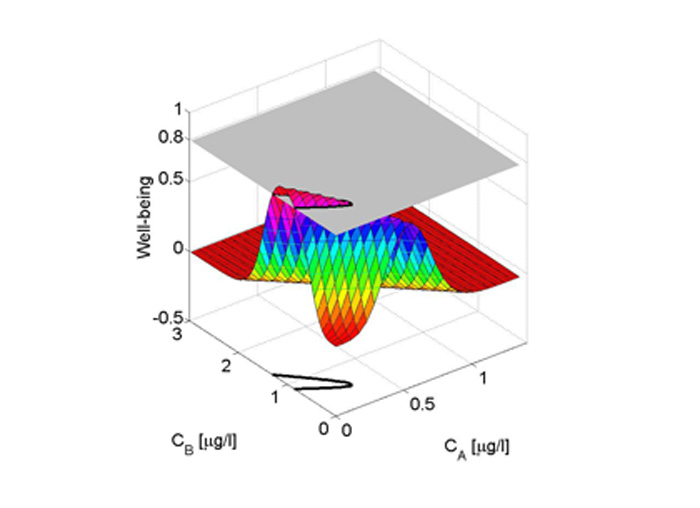
The main advantage of the proposed extension is that, by including the limitations imposed by the negative effects into the expression for the well-being function, the "optimal dosage" can be directly defined as the one generating effect compartment concentrations lying in a range as to lead to the maximum well-being value. This is the set, which should be targeted by drug infusions. Graphically, this set is obtained by projecting on the concentrations plane the intersection of the well being surface with a plane at the defined minimal level of adequate well-being. This modeling framework improves our understanding of the characteristics of drug combinations used in clinical practice and can be used in clinical research to identify optimal drug dosing.
[Paper]
Optimal Experiment Design
The pharmacokinetic (PK) describes the time course of drug concentration in the body. Mammillary pharmacokinetic models represent the human body through a limited set of compartments, which have usually no physiologic or anatomic reality. These models are widely used to design Target Controlled Infusion Pumps (TCI) commonly used by anesthetists in clinical practice.
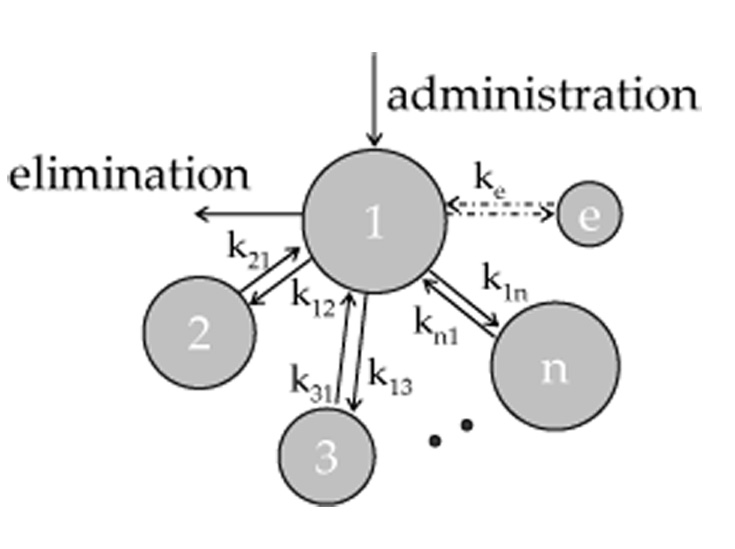
The experimental setup of pharmacokinetic study plays a critical role in the parameter estimation process: depending on the sampling time and on the administration mode different parameter set can be obtained for the same mammillary model. A poor estimation of the model parameters, in particular of the central volume of distribution, will determine a low quality of the model predictions. As direct consequence, the implementation of such model in a TCI system could result in overdosing the patient.
The optimal parameter set can be identified as the one that, implemented in a TCI system, will provide concentration minimally deviating from the target.
The aim of this work is to find out how an experiment should be designed in a way of optimally estimate the parameters of a pharmacokinetic mammillary model.
On-line Parameter Estimation
Based on pharmacokinetic and pharmacodynamic modeling of drug action and interactions, an advisory system (Anesthesia Advisory Display, AAD) has been recently proposed [Schumacher et al., ASA 04, Las Vegas]. This system comprises a two dimensional "effect map" described as a function of the two effect compartment concentrations of the hypnotic agent propofol and of the opioid remifentanil. While leaving the anesthetist in complete control of dosing (the physician directly manipulates the infusion pumps), the display system reads the current infusion rates and, using the implemented pharmacokinetic-pharmacodynamic model, displays in an intuitively appealing format the actual predicted drug concentrations, the predicted combined effect and predicted wake up time resulting from his actions in the typical patient. These predictions are dynamically updated according to the dosing history. Therefore, possible suggested uses are training and online use in the OR.
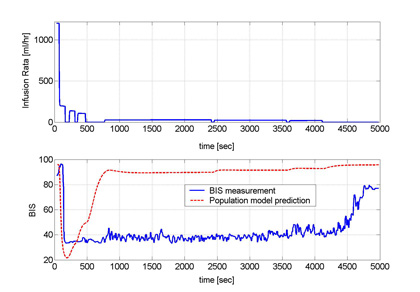
The use of a combined pharmacokinetic-pharmacodynamic (PKPD) model to determine the dose regimen to administer to a patient is complicated by the large patients inter-variability. Therefore the implementation of a population PKPD model in the AAD can lead to a mismatch between model prediction and real measurement. The occurrence of a large mismatch will decrease the usefulness of the advisory system, undermining the confidence of the physician in such a system, therefore limiting its use. As an example, this mismatch will be apparent when considering unconsciousness, expressed through the measurable index BIS (Aspect Medical Systems Inc., Newton, MA), as shown in Figure 1.
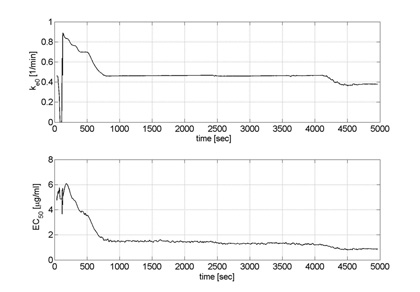
To improve the prediction information for BIS displayed in the AAD, a model parameter adaptation strategy for propofol based on the Kalman filter algorithm has been developed. The method has been tested on the data of 80 patients from a previous clinical study.
Figure 2 shows the estimated parameters for patient No. 24. After an initial transient time, the parameters converge to a steady value. To assess the prediction performances of the adpated model, at each time step the last available estimates of the parameters were fed into the PKPD model to predict the value of BIS after an interval DeltaT, according to the infusion rate of propofol administered to the patient. The prediction errors of the adapted model were significantly smaller than the prediction errors of the population model (p<0.01). [Paper]